The Kuhn Tucker Conditions are a fundamental aspect of optimization, particularly in the field of mathematical programming. These conditions serve as necessary and sufficient criteria for optimality in problems that involve inequalities and constraints. In the realm of economics, engineering, and operations research, grasping the Kuhn Tucker Conditions is crucial for formulating effective solutions to complex decision-making problems. They help identify the best possible outcomes when faced with limitations, guiding analysts and researchers in making informed choices. By setting the stage for optimization, the Kuhn Tucker Conditions have become a cornerstone in various domains, showcasing their significance in both theoretical frameworks and practical applications.
Understanding the Kuhn Tucker Conditions not only requires a grasp of the underlying mathematical principles but also an awareness of how these conditions interact with real-world scenarios. With the rise of computational methods and algorithmic approaches, the application of these conditions has become increasingly prominent, allowing for the resolution of complex optimization problems that were once deemed insurmountable. Consequently, the relevance of the Kuhn Tucker Conditions continues to expand as industries evolve and new challenges emerge.
As we delve deeper into the nuances of the Kuhn Tucker Conditions, it is essential to explore their components, implications, and applications. This article aims to demystify these conditions, providing insights into their role in optimization and offering practical examples of their use in various fields. From understanding the mathematical foundations to exploring real-world applications, we will navigate through the intricacies of the Kuhn Tucker Conditions, equipping readers with the knowledge to leverage this powerful tool in their endeavors.
What Are the Kuhn Tucker Conditions?
The Kuhn Tucker Conditions, originating from the work of Harold W. Kuhn and Albert W. Tucker in the 1950s, provide a set of conditions that must be satisfied for a solution to be optimal in a constrained optimization problem. These conditions extend the earlier Lagrange multipliers method, which is applicable to equality constraints, to accommodate inequality constraints as well. The conditions are essential in both theoretical mathematics and practical applications, forming a bridge between the two fields.
How Do Kuhn Tucker Conditions Work?
The Kuhn Tucker Conditions consist of several components, including primal feasibility, dual feasibility, and complementary slackness. In essence, they ensure that not only are the constraints satisfied but also that the solutions are optimal concerning the objective function. Here's a brief overview of each component:
- Primal Feasibility: This condition requires that the solution satisfies all the original inequalities and equalities of the problem.
- Dual Feasibility: This involves ensuring that the multipliers associated with the constraints are non-negative.
- Complementary Slackness: This condition states that for each inequality constraint, either the constraint is active (satisfied as an equality) or the associated multiplier is zero.
Why Are Kuhn Tucker Conditions Important?
The importance of the Kuhn Tucker Conditions lies in their ability to provide a systematic approach to solving complex optimization problems. In fields such as economics, engineering, and operations research, these conditions allow practitioners to:
- Identify optimal solutions under constraints.
- Analyze the sensitivity of solutions to changes in constraints or parameters.
- Develop algorithms for numerical optimization.
What is the Relationship Between Kuhn Tucker Conditions and Lagrange Multipliers?
The Kuhn Tucker Conditions can be viewed as an extension of the method of Lagrange multipliers, which is used for optimization problems with equality constraints. While Lagrange multipliers handle only equalities, Kuhn Tucker Conditions address both equalities and inequalities. This broader applicability makes the Kuhn Tucker Conditions essential for more complex optimization scenarios.
How Are Kuhn Tucker Conditions Applied in Real-World Scenarios?
The applications of the Kuhn Tucker Conditions are vast and varied, spanning multiple industries. Here are some notable examples:
- Economics: In economic models, the conditions help determine optimal production levels while considering resource constraints.
- Engineering: Engineers use these conditions to optimize designs, ensuring performance meets safety and regulatory constraints.
- Operations Research: Businesses apply the Kuhn Tucker Conditions to optimize logistics and supply chain operations.
Can Kuhn Tucker Conditions Be Visualized Graphically?
Yes, the Kuhn Tucker Conditions can often be visualized using graphical representations, particularly in two-dimensional spaces. By plotting the feasible region defined by the constraints, one can observe the optimal solution at the intersection of the objective function and the boundary of the feasible region. This visual representation aids in understanding how the conditions interact and provides an intuitive grasp of optimization.
What Challenges Are Associated with Kuhn Tucker Conditions?
Despite their utility, there are challenges associated with the Kuhn Tucker Conditions. Some of these include:
- Complexity: As the number of constraints increases, the conditions become more complex, making them harder to solve analytically.
- Non-Convex Problems: In non-convex optimization problems, the Kuhn Tucker Conditions may provide multiple local optima, complicating the search for a global solution.
- Computational Resources: Solving large-scale optimization problems using the Kuhn Tucker framework can require significant computational resources.
What Future Developments Can Be Expected in Kuhn Tucker Conditions?
As optimization problems become increasingly complex, future developments in the Kuhn Tucker Conditions may focus on enhancing computational efficiency and expanding their applicability. Researchers are likely to explore advanced algorithms and heuristics that can streamline the solution process, making it feasible to tackle larger and more intricate problems. Additionally, interdisciplinary approaches may emerge, integrating insights from fields like machine learning and artificial intelligence to further refine optimization techniques.
Conclusion: Embracing the Kuhn Tucker Conditions in Optimization
The Kuhn Tucker Conditions represent a vital aspect of optimization theory, enabling practitioners to navigate the complexities of constrained problems effectively. By understanding and applying these conditions, individuals in various fields can derive optimal solutions that align with their constraints and objectives. As industries continue to evolve and confront new challenges, the relevance of the Kuhn Tucker Conditions will undoubtedly grow, solidifying their place as a fundamental tool in the optimization toolbox.
Article Recommendations
- Anime Character Male
- Glenn Close Michael Douglas
- Cnn What Does Donal Trump Want To Do With Violence
- When Did Confessions Come Out
- Birth In Bathroom
- New Year Movie 2024
- Kelsey Lawrence And Dabb Fan Bus Video
- Ribcage Straight Ankle Jeans
- Cast From The Secret Life Of The American Teenager
- Who Is The Song Youre So Vain About
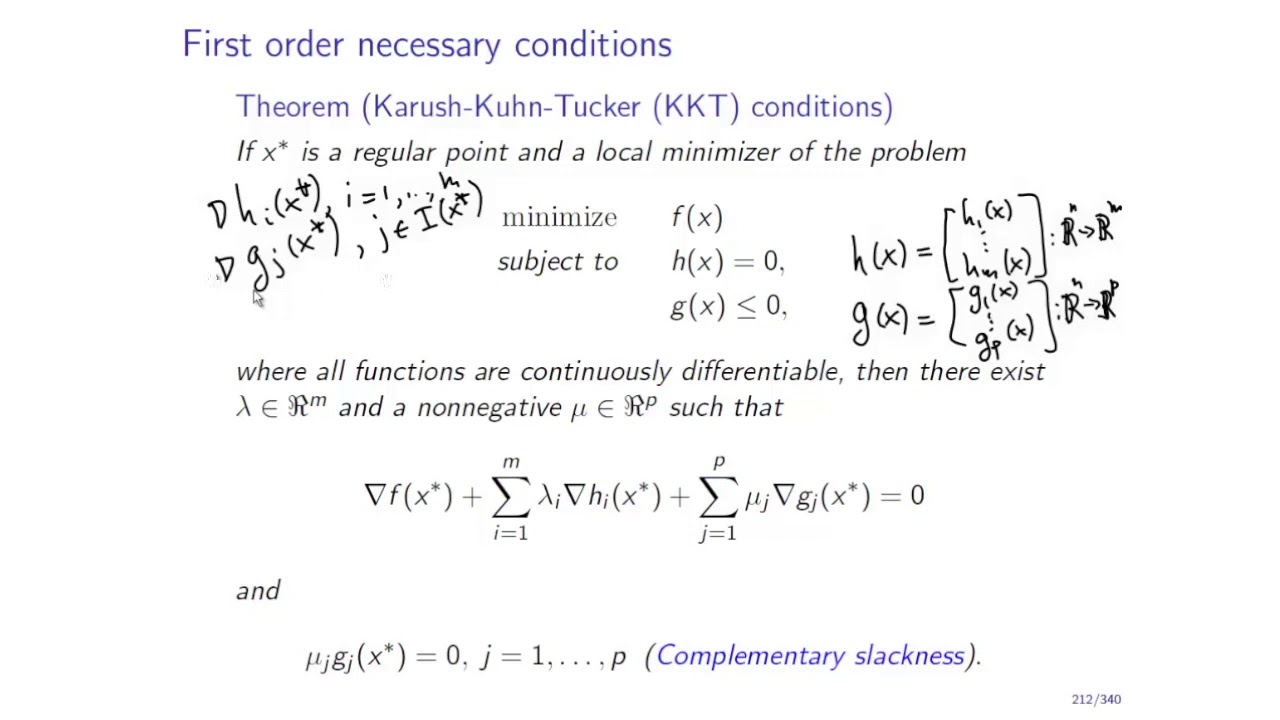
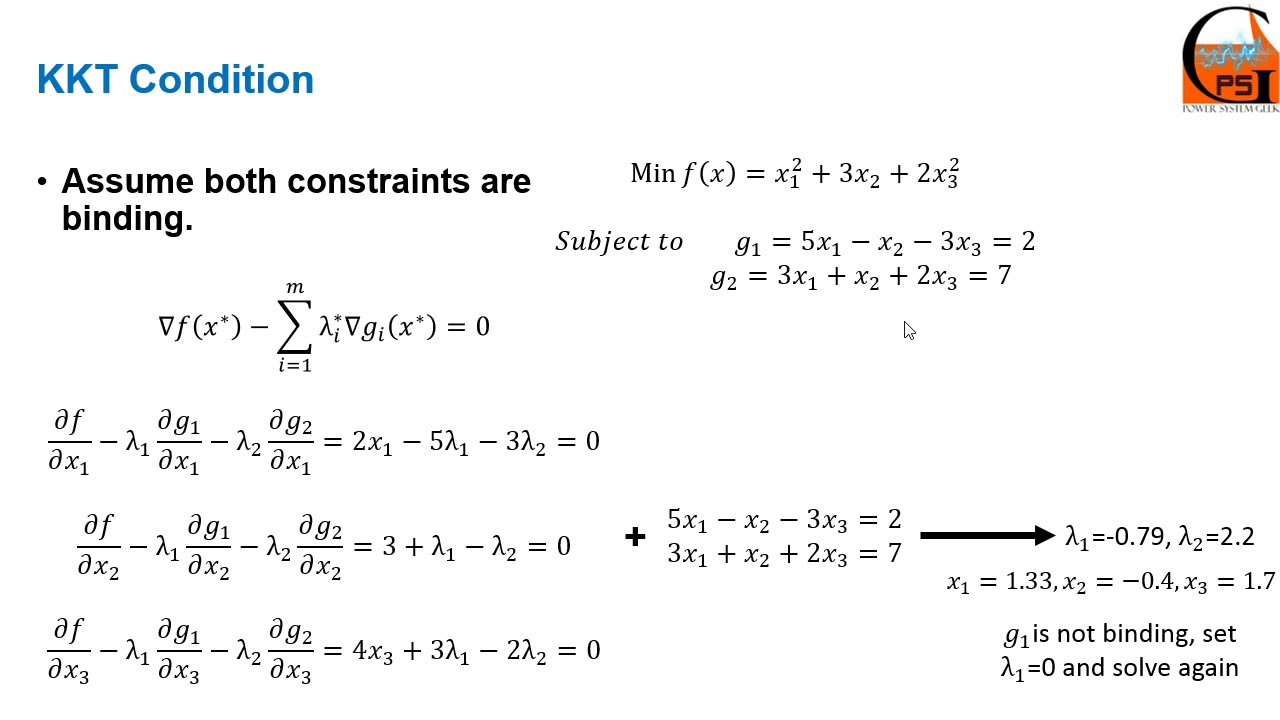
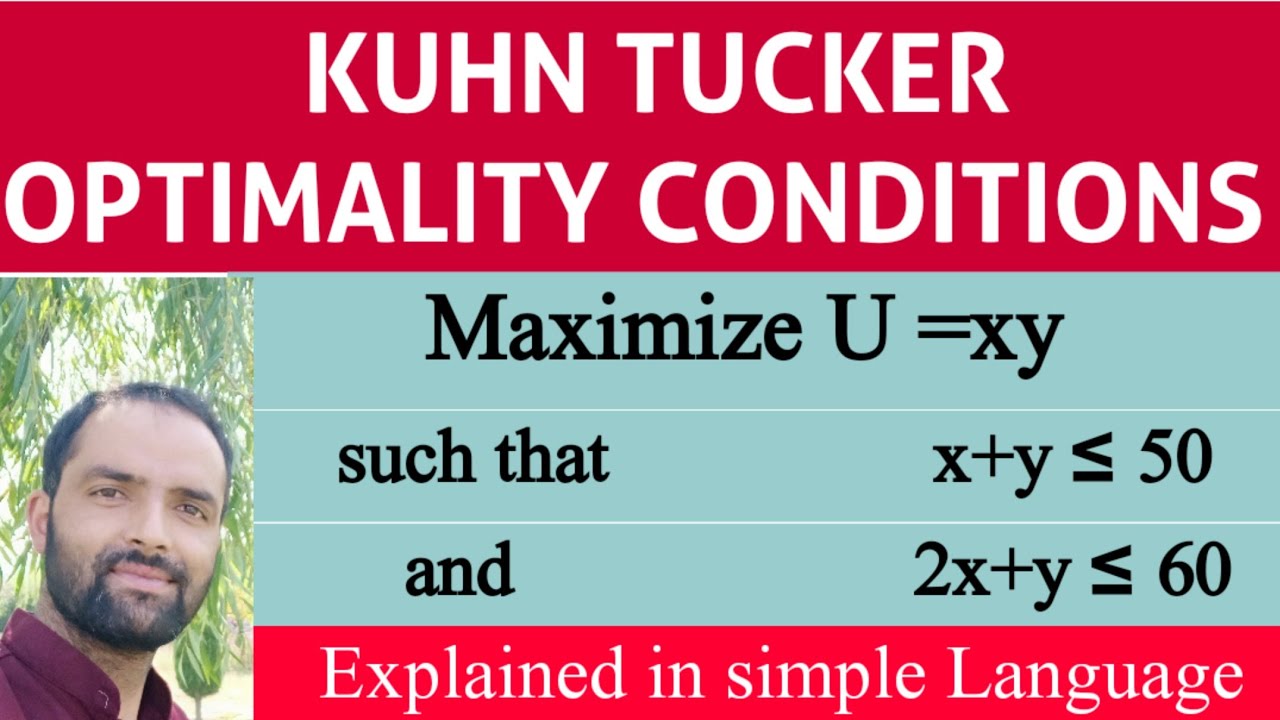